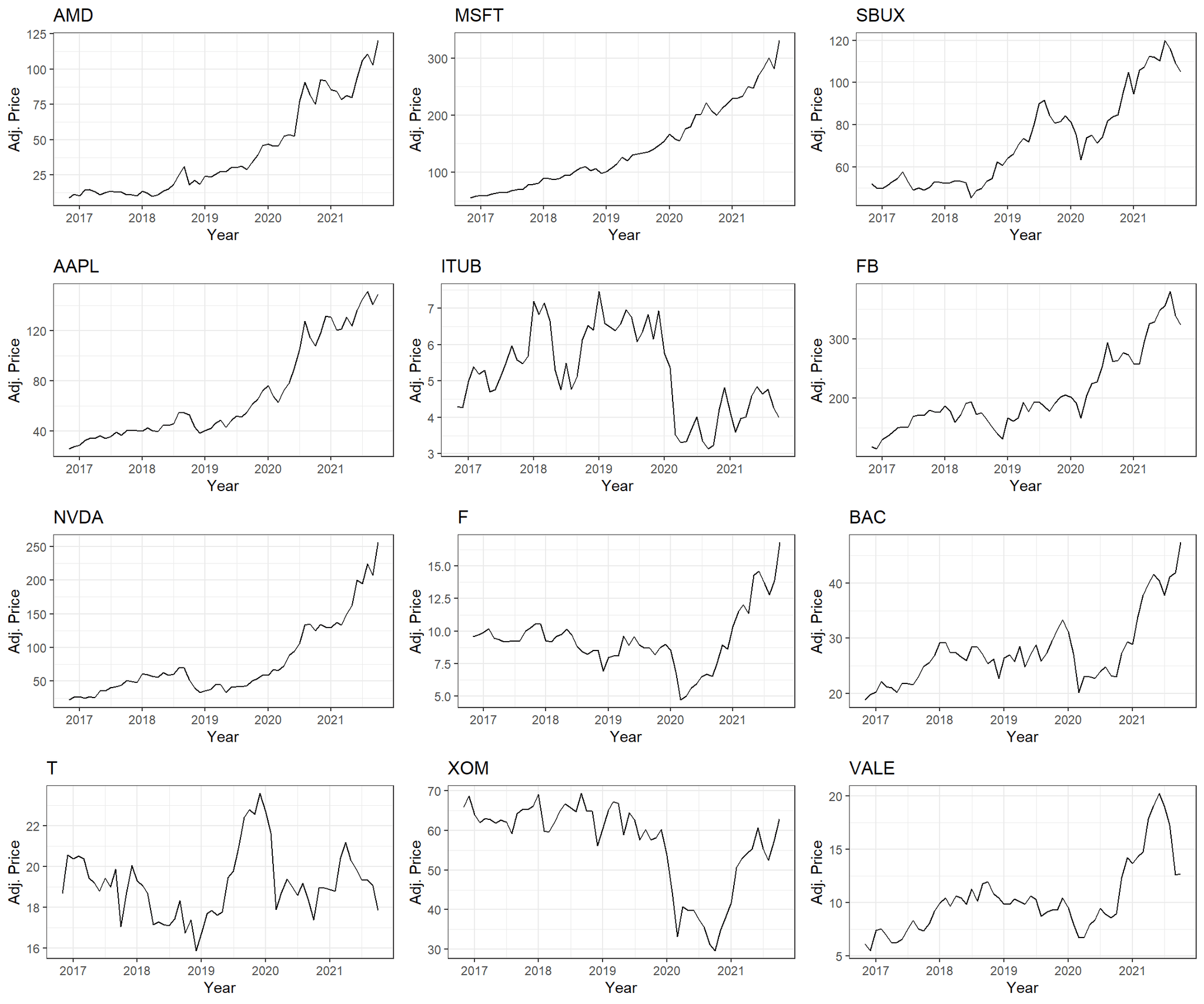
Statistical Insights into Diversified Portfolio Analysis: Unveiling Financial Trends and Strategies
Financial analysis on big-tech companies stocks until 2021 using statistical financial methods.
Introduction: The project centered on analyzing a diverse portfolio comprising stocks from the technology sector and various other industries. This approach aimed to evaluate the risk-return profile of each asset using advanced statistical techniques, providing valuable insights into their behavior over time.
Key Findings:
1. Non-Normal Distributions: One of the prominent discoveries was that the majority of assets in the portfolio did not adhere to normal distributions. Instead, they followed Skewed Standardized-t distributions, with some fitting a Generalized Error Distribution pattern.
2. Performance Insights: Among the analyzed assets, Microsoft emerged as a standout performer with consistently high returns and a robust reward-to-risk ratio. This highlighted Microsoft's resilience and profitability within the portfolio context.
3. Asset Independence: A significant observation was the near-zero covariance among many assets. This finding indicated a level of independence between assets, underscoring the importance of diversification in minimizing portfolio risk effectively.
4. Copula Analysis: Employing t-Copula modeling, the study revealed an increased likelihood of joint extreme events among asset values. This aspect emphasized the necessity of robust risk management strategies to mitigate potential losses during market downturns.
5. Portfolio Construction: Various portfolio strategies were explored, including the Minimum Variance Portfolio (MVP) and Efficient Portfolio, aiming to optimize returns while managing risk levels prudently. These strategies underscored the application of statistical methods in constructing balanced and efficient investment portfolios.
Methodology:
- Data Collection: Historical monthly prices and returns data spanning a five-year period were collected for stocks such as AMD, Microsoft, Apple Inc., Meta Platforms Inc., and others.
- Statistical Techniques: The project employed a range of statistical tools including Q-Q plots, boxplots, and hypothesis testing to validate assumptions and derive meaningful insights from the data.
- Risk Management: Techniques like Value at Risk (VaR) and Expected Shortfall (ES) were utilized to quantify and manage potential financial risks associated with the portfolio holdings.
Conclusion: The project exemplifies the critical role of statistical methods in financial analysis, providing actionable insights into asset performance, risk assessment, and portfolio optimization. These insights are invaluable for investors seeking to navigate the complexities of modern financial markets with confidence and precision.
Future Directions: Future research endeavors could expand on this analysis by incorporating a broader range of assets or integrating machine learning algorithms for predictive modeling. Such advancements could further enhance portfolio management strategies, offering deeper insights and improved decision-making capabilities.
Conclusion: In summary, the Statistical Methods In Finance final project offers a comprehensive exploration of portfolio dynamics and performance metrics, demonstrating the power of statistical rigor in illuminating financial trends and informing investment strategies. By leveraging these insights, investors can effectively manage risks and capitalize on opportunities in today's dynamic financial landscape.